Understanding Value-at-Risk (VaR)
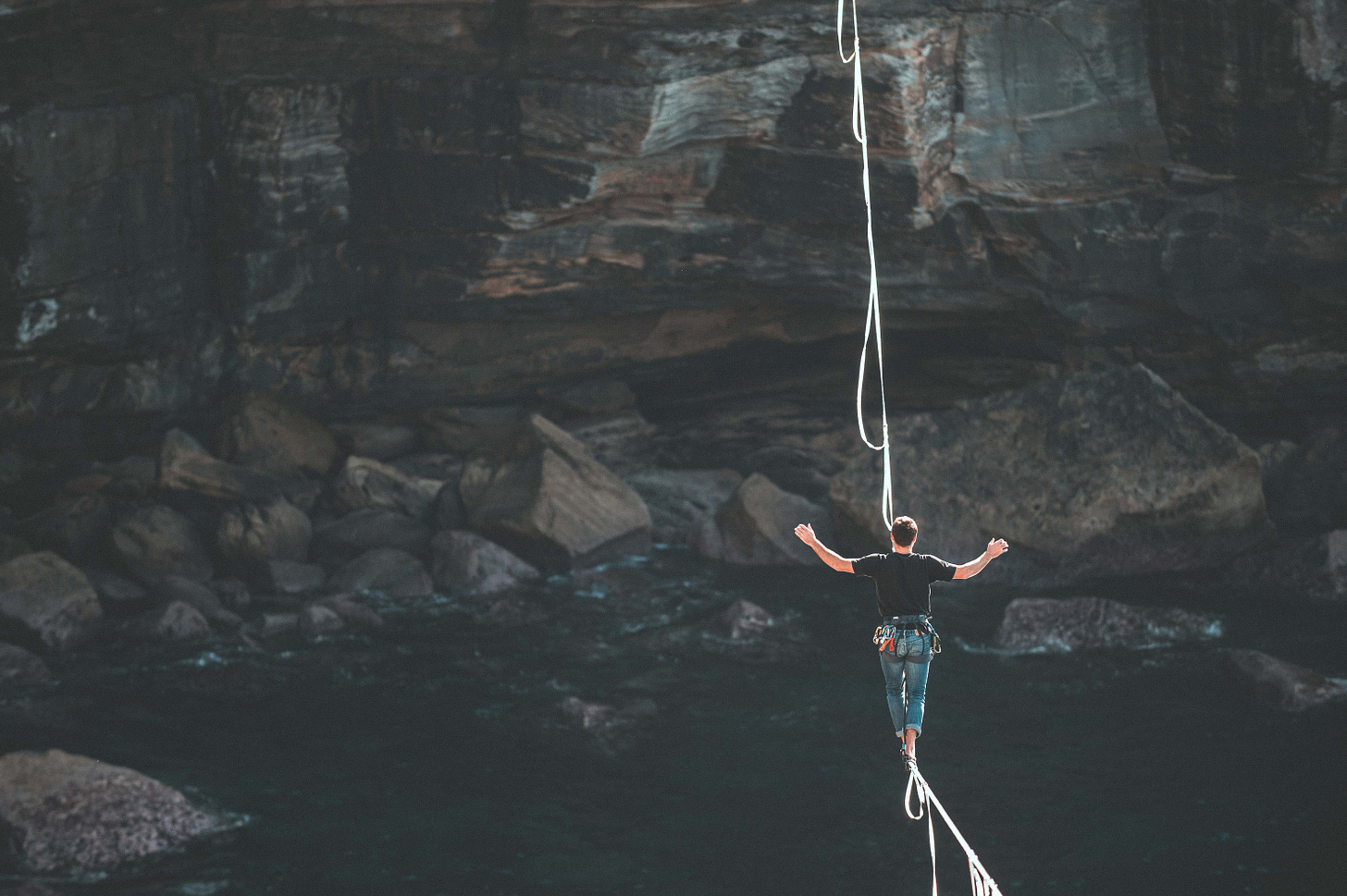
VaR stands for Value-at-Risk. It’s a hugely important component of any form of trade because it is a straightforward method to quantify the risk of a single asset or entire portfolio at any point in time. Using VaR modelling, we can define the likelihood of losses in a portfolio and use this to create different collections of assets that represent risky and safe sentiment. If we’re feeling bullish on certain economies, we can begin to invest in assets from our risky pool. If you believe the economy is headed towards a downturn, we may look to safer investments. With VaR, we can constantly keep an eye on how the probability of losses and extent of losses dynamically changes as we introduce and remove different assets.
This article is going to outline the use of VaR as a method of applying a Stop Loss (a price in the opposite direction of our placed trade to manage our loses if the market goes against us) for a trade on a daily timeframe, but it will work on any timeframe you want to use. This method is credited to David Carli and his book Forex Fundamental Analysis, which is where I found the concept being discussed for this purpose.
Calculations
Before we jump into exactly how to use this method, we need to address how VaR is represented. Typically the VaR of an asset or portfolio is the “y% that said asset/portfolio will fall by at least x% over a defined time period”. For example, we might say that, using our analysis on the EURUSD daily timeframe, we have calculated that this currency pair has a 5% chance of falling by at least 2.12%. This figure can serve as a confidence interval and so if we decided to buy EURUSD, we could set our stop loss at 2.12% below the buy price, a figure that we believe the market only has a 5% chance of reaching.
There are multiple methods through which we can determine this percentage. This article is going to cover the historical method. Essentially we are going to use historical prices to find the “anomalous” daily percentage changes and set the minimum of these values as our VaR-modelled Stop Loss figure. The calculation of this in Excel is as follows:
Download a historical daily prices dataset
Calculate the percentage change for the daily closing prices -> (current row - previous row)/ previous row or (B3 - B2)/B2
Copy these values to an adjacent column, because we cannot order a calculated field
Sort this field in ascending order
Calculate how many rows are required for your confidence intervals. This is done by taking the number of rows in your dataset, and multiplying it by 100 - CI, where CI is your confidence interval. For example, say we have one year of data, 252 business days and we want to calculate a 95% confidence interval. We would calculate this as 252 * (100 - 95) = 12.6 ≈ 13
For the 95% confidence interval, take the absolute % change value at the 13th row as your VaR Stop Loss figure
I ran this calculation on the major and minor forex pairs for 1 year’s worth of data until the closing price last week, assuming symmetry among the positive and negative % changes, to generate a 95% upper and lower bound confidence interval for the daily timeframe. To run you through an example scenario for how to use this, let’s say we wanted to place a buy trade on GBPUSD as soon as the markets opened Monday morning. Our most recent close is 1.3899 so let’s assume that’s the price we would get had we bought on Monday. We can use the lower bound as our Stop Loss, which is 1.3775, 0.89% below the most recent close. Based on historical performance, we believe there is a 95% chance the GBPUSD will not fall to this level over the course of trading on Monday.
There are few issues with this method of calculation. Naturally, it’s quite a crude method of calculating a Stop Loss, because it relies on past behaviour being linked to future behaviour. Whilst we can’t assume this to be true, what we do assume is that with enough data points, we can still identify anomalous market days and use this to estimate bounds for our prices. It’s the large amount of data points that we use that helps us to provide more accurate bounds, because whilst we can’t predict exact daily behaviour, we can create a framework that identifies anomalous performance and use this to structure our trades.
If you were to place a trade on a day in which a major economic announcement occurred, however, then the quality of the bounds might be less rigid, since highly unexpected announcements have the ability to wildly swing the markets. It’s why most professional investors recommend that retail traders avoid trading on announcement days, since these have the power to stop you out of a trade very quickly.
It’s important to note that this method will not tell you which way a market it expected to move over the course of a day. Just because we have an upper and lower bound doesn’t mean that we can place either a buy or a sell. Our economic investigations still have to be carried out along with the timing of actually entering a trade. The purpose of VaR in this example is purely to help us understand what kind of Stop Loss might give us a fighting chance of pulling off a successful trade, assuming our other analysis is correct.