Introducing Random Walk Models
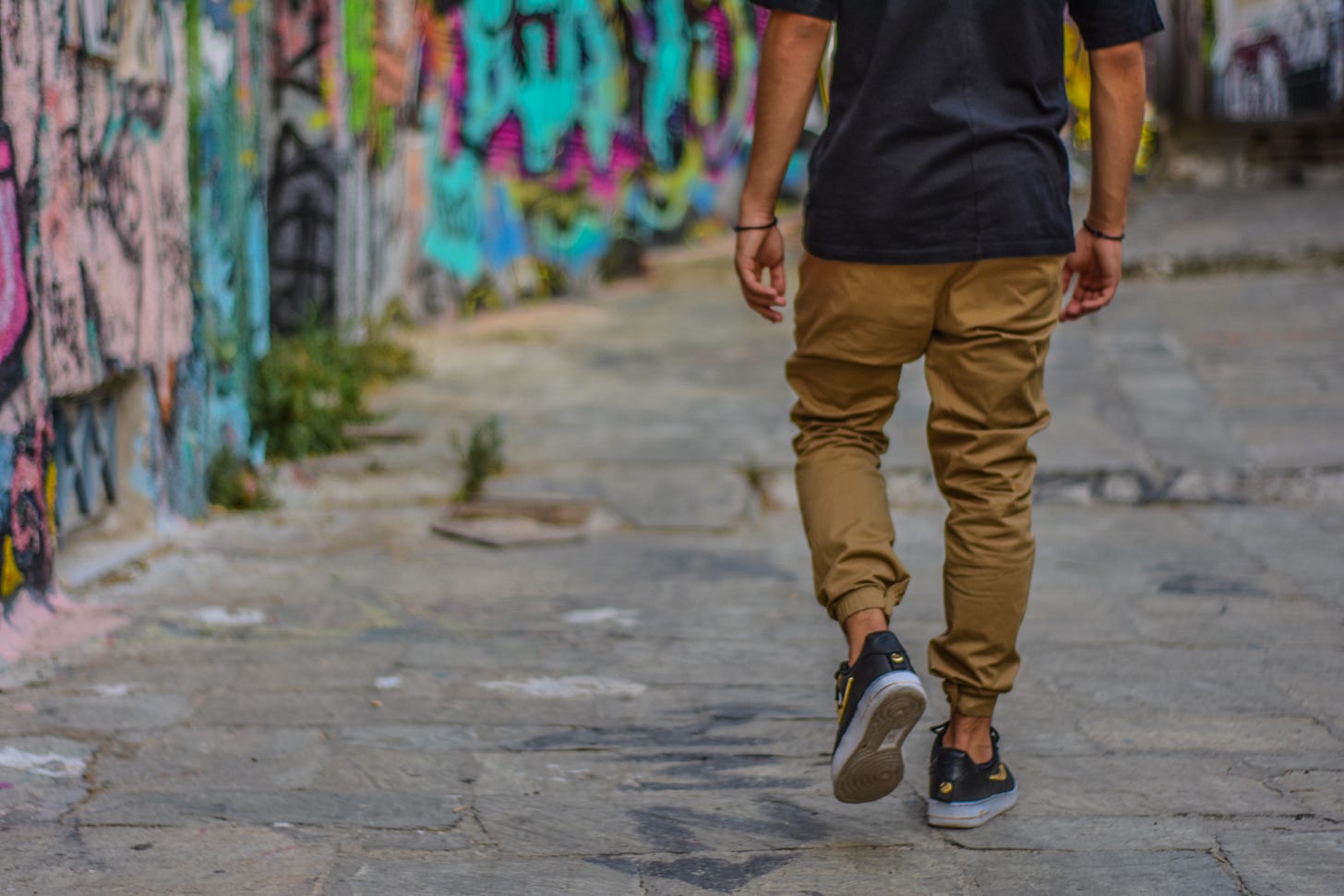
The broad goal for time series analysis is to be able to build a model that identifies all autocorrelation in a time series and uses this to create trend forecasts. We want to be able to use all the information from past behaviour that we believe is relevant to future movements with the hope that all is left is to add some form of uncorrelated error function that is derived from a common distribution. Whilst, especially in the financial markets, this level of accuracy is more an optimal/un-reachable solution than an attainable one due to the many other factors at play, the principle goal is still something we can work towards. With time series analysis, we alter the above to ask the following question -> how much of past behaviour can we use to model future prices and what external factors and error components can we add to model what’s left?
One of the starting points for any time series analytics is to produce a random walk model. Whilst they can vary in intelligence depending on how much information you provide to determine the direction they should be moving in, random walks models provide a robust framework to different types of predictions. We can use a random walk model to create a benchmark against which we grade other, more sophisticated models we use in the future. We could also use this model as the sophisticated model itself, by providing intelligence to determine probabilities of price moving up or down.
Theory
One benefit of random walk models is that the underlying theory is relatively straightforward. In a sentence, a random walk model will create a new observation by taking the previous one and adjusting it with a random step up or down. Typically, this random step is the residual value, the error that occurs in our model that we would hope are independent and identically distributed variables since we have already abstracted away as much of the autocorrelation as we can. These values are therefore small values such that they don’t explain large shifts in the data that we would expect to come from an external macro cause. We can also see that we maintain the correlation of our time series by using the previous observation as a starting point for our forecast.
Building Models
If we decided to make this model purely random i.e. 50% chance of moving up at each step, 50% chance of moving down, we have a benchmark. We can measure other models against this to see if they perform better that one without any intelligence. To provide an example of a completely “random” random walk model, I have outlined a strategy below.
Building intelligence into this model is reflected in the probability that we assign to a step up or down. If we know that there’s a higher likelihood of the price moving in a certain direction, it follows logic that we should alter our probabilities. The use of probabilities means that we can also account for the price moving in the opposite direction to what we might expect.
Assigning new probabilities can come from a variety of sources and is not s a straightforward task. We can use historical behaviour i.e. number of steps up or down in the past x observations as a proxy for our probabilities. We could also use macro observations e.g. whenever the government has reduced interest rates by over 1% over the past 20 years, the following week has seen the currency rate for that country fall in 6 out of the 10 instances, therefore making our probability of a step down 60%. We can also use a combination of many features, each of which will have its own individual weightage toward the probabilities, likely to be calculated through some form of Linear Regression.
The following dashboard provides a simple hypothetical example, in which, from previous behaviour, we have determined the percentage of a step up or down during a change in the interest rates in a currency. Note here that we also introduce another method of calculating the value of our step. It’s important to consider this parameter aswell. We might know the direction we expect prices to travel, we might also know the value we expect a currency pair to rise or fall to, but we also need to determine how quickly it gets there. Prices in the market don’t move by the same amount every day and so some intelligence also needs to be incorporated into the calculation of these steps. An alternative method to the one described below might be to calculate a range of expected values and use a random generator within that range to determine the step value for that particular time period.
This is just an introduction to Random Walk Models, but I hope you can see that there is a huge scope to work with this framework and build models that range in complexity. Random Walks allow you to incorporate a significant amount of external behaviour into its forecasts and this is hugely beneficial when studying time series as complex and dimensional as those from the financial markets. I’m currently working on building some prediction dashboards that I will make available to view as soon as they are ready and you can be sure to find some highly involved random models in use there for the forecasts.
As a final note before I wrap up this article, I wanted to include a not-so-subtle attempt at plugging this newsletter I’m running. I would appreciate any help in spreading these articles and to help Algo-Fin continue to gain traction. I’m still working on launching the paid side of my newsletter, but regardless of this, my weekly articles covering time series modelling, machine learning techniques and market philosophy will continue for as long as this newsletter is in operation. If you know someone that might be interested in this content, feel free to share it with them or on any social media site you’re on. I’m hugely appreciative of all your support so far and will continue to be as this newsletter continues to grow!